Advertising and marketing experimentation can function a aggressive benefit by enabling organizations to develop quicker than their friends, based on a complete information launched by Google. The doc outlines how firms can construct what Google phrases a “measurement muscle” via systematic testing of selling actions.
The worth of this experimental method manifests in concrete numbers. Based on Google’s evaluation, organizations conducting month-to-month experiments with 5% effectiveness enhancements obtain a 3.1x improve in returns over a two-year interval. Firms operating quarterly experiments see a extra modest however nonetheless important 1.4x enchancment in the identical timeframe.
“Advertising and marketing and promoting, if executed effectively, can function a aggressive benefit for any firm,” states the information. “However how can we decide whether or not advertising is being executed effectively? That is the place experiments are available.”
The doc emphasizes that experimentation allows organizations to maneuver past correlation to determine causality in advertising effectiveness. This functionality proves essential for answering elementary questions on media efficiency, together with optimum funds allocation, viewers focusing on, and artistic technique.
To construct this experimental functionality successfully, Google recommends assembling what it calls an “experimentation squad.” This workforce requires 4 distinct ability units: a artistic strategist to generate testing concepts, a enterprise analyst to prioritize experiments based mostly on potential impression, an information scientist to make sure statistical validity, and a decision-maker to allocate assets and implement findings.
The methodology emphasizes 4 essential traits of high-quality experiments. First, experiments should hyperlink inquiries to particular actions. The information warns in opposition to conducting exams purely for data gathering, stating that each experiment ought to hook up with concrete enterprise choices.
Second, experiments have to deal with significant metrics that tie on to enterprise targets. Google introduces an effectiveness-efficiency framework that balances progress indicators in opposition to value constraints. This method helps organizations maximize returns whereas sustaining profitability targets.
Statistical concerns type the third pillar of the methodology. The information emphasizes the significance of correct experimental design, together with energy evaluation to find out sufficient take a look at period and pattern sizes. This mathematical rigor helps organizations keep away from drawing incorrect conclusions from inadequate information.
Lastly, experiments should account for broader context. The information cautions in opposition to over-generalizing outcomes, noting that advertising effectiveness varies throughout time durations, geographical areas, and financial situations. This understanding drives the advice for normal testing cycles fairly than counting on historic information.
For organizations starting their experimental journey, the information recommends beginning with clear enterprise questions linked to organizational key efficiency indicators. This method ensures that experimental findings translate straight into actionable insights that drive enterprise progress.
The doc additionally introduces the idea of metric waterfalls, which assist organizations deal with information sparsity challenges. This framework allows firms to make knowledgeable choices even when main metrics lack ample information by incorporating correlated secondary and tertiary indicators.
The methodology emphasizes that constructing experimental functionality requires sustained dedication. Based on Google, the compound impact of normal testing creates a “aggressive benefit that permits them to develop extra shortly than their friends.”
This systematic method to experimentation represents a shift from conventional advertising measurement. Slightly than counting on correlation-based analyses or periodic effectiveness research, organizations can construct steady studying capabilities that drive ongoing optimization of selling investments.
The information concludes by highlighting the dynamic nature of selling effectiveness, emphasizing that experimental outcomes stay legitimate solely inside particular contexts. This actuality underscores the significance of constructing strong experimental capabilities that allow organizations to adapt quickly to altering market situations.
By this methodical method to experimentation, organizations can develop what Google describes as a “measurement muscle” that gives sustainable aggressive benefit in more and more advanced advertising environments.
Understanding the KPI waterfall: An information-driven method to advertising measurement
The problem of measuring advertising effectiveness typically lies in information shortage, an issue Google addresses via what it calls the “KPI waterfall” framework, based on its experiments playbook. This structured method helps organizations stability the competing calls for of statistical significance and enterprise relevance.
On the coronary heart of this technique lies a elementary problem in advertising measurement. As said within the information, main enterprise metrics, akin to income or buyer acquisitions, ceaselessly happen too occasionally to offer statistically important outcomes inside cheap testing timeframes.
Google illustrates this idea via a case examine of an automotive firm. Based on the doc, automotive purchases characterize the last word enterprise end result however happen too hardly ever to function dependable experimental metrics. The corporate wanted to establish various measurements that would point out effectiveness whereas sustaining ample information quantity for statistical validity.
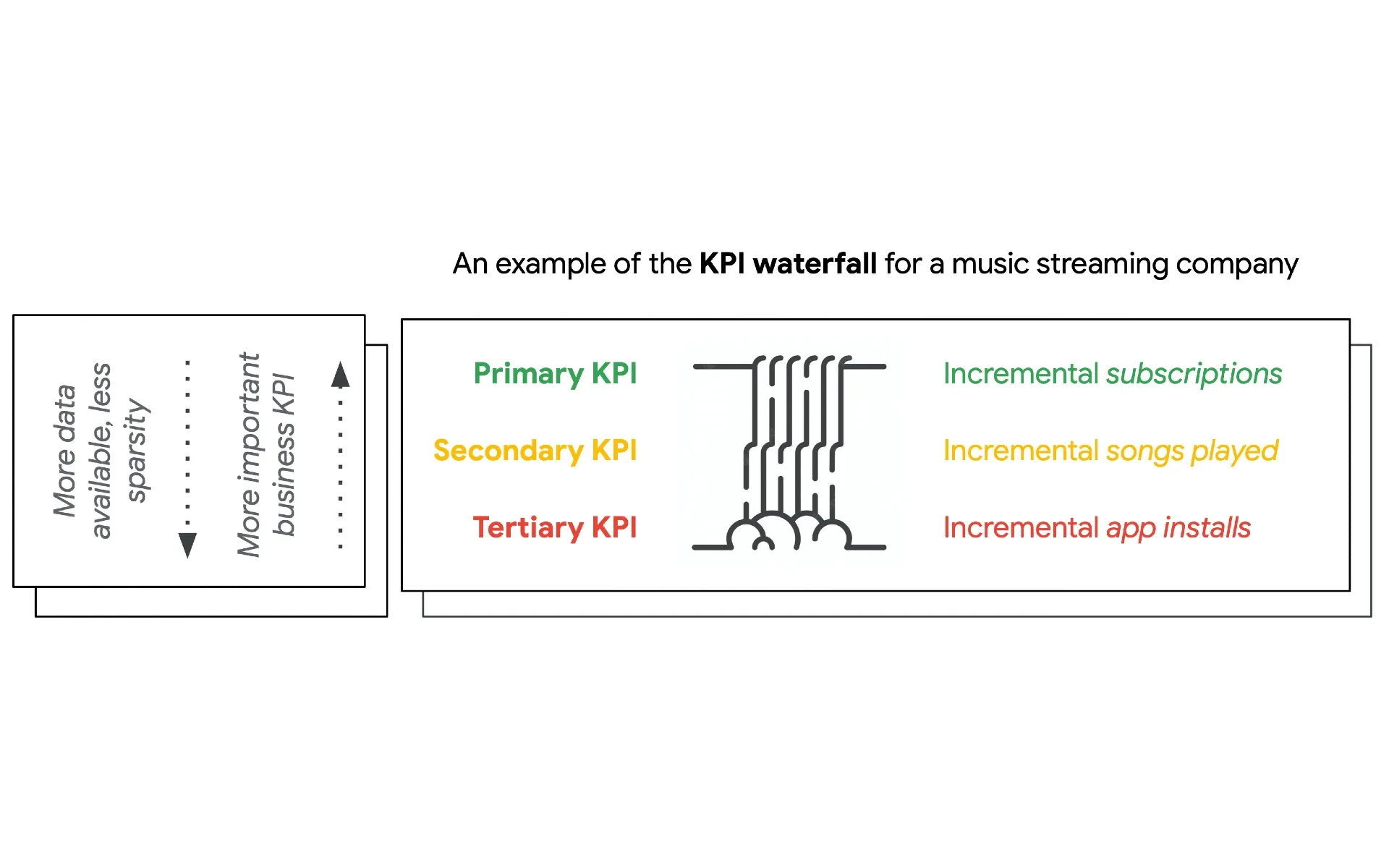
KPI waterfall framework
The KPI waterfall framework addresses this problem via a three-tiered metric system. Based on the information, every tier serves a particular function whereas sustaining clear connections to enterprise targets. A music streaming service instance demonstrates this hierarchy: subscriptions function the first KPI, songs performed because the secondary metric, and app installs because the tertiary indicator.
The methodology emphasizes the connection between metric frequency and enterprise significance. Larger-tier metrics sometimes carry higher enterprise significance however happen much less ceaselessly. Decrease-tier metrics generate extra information factors however could have much less direct enterprise impression. The framework helps organizations navigate this trade-off systematically.
Google gives an in depth instance via ChewyTreats, an internet pet food retailer testing totally different video artistic approaches. The experiment tracked three metrics: incremental conversions, add-to-cart actions, and web site visits. When main and secondary metrics confirmed directionally constructive however statistically insignificant outcomes, the extra frequent tertiary metric of web site visits supplied actionable insights.
The framework’s sensible software turns into evident within the outcomes evaluation. Based on the doc, ChewyTreats’ incremental conversions confirmed ranges of [12k-16k] versus [13k-17k] between take a look at teams, whereas web site visits demonstrated clear statistical separation at [100k-130k] versus [140k-165k]. This information quantity enabled assured decision-making regardless of main metric uncertainty.
Essential to the framework’s success is the correlation between metric tiers. The information emphasizes that lower-tier metrics should exhibit sturdy relationships with main enterprise outcomes to function legitimate proxies. Organizations have to validate these correlations earlier than incorporating metrics into their measurement framework.
The methodology additionally addresses the temporal side of selling measurement. Based on the information, higher-funnel metrics typically present earlier indicators of marketing campaign efficiency, enabling quicker optimization cycles whereas sustaining alignment with final enterprise targets.
Google’s framework consists of particular steering for metric choice. The doc recommends selecting secondary and tertiary metrics that stability three key standards: information availability, enterprise relevance, and confirmed correlation with main outcomes. This structured method helps organizations keep away from the pitfall of optimizing for simply measured however commercially irrelevant metrics.
The KPI waterfall framework represents a major development in advertising measurement methodology. By systematically incorporating metrics of various frequency and enterprise impression, organizations can keep each statistical validity and industrial relevance of their experimental packages.
By this structured method to metric choice and evaluation, firms can construct extra strong measurement capabilities whereas staying centered on final enterprise outcomes. The framework gives a sensible resolution to the persistent problem of knowledge sparsity in advertising experimentation.

Source link