Enter from theCUBE and knowledge practitioner communities means that acceleration in compute efficiency and the sophistication of the fashionable knowledge stack is outpacing the wants of many conventional analytic workloads.
Most analytics workloads at this time are carried out on small knowledge units and usually can run on a single node, considerably neutralizing the worth of distributed, extremely scalable knowledge platforms. As such, we consider at this time’s trendy stack, or MDS, which began out serving dashboards, should evolve into an clever utility platform that harmonizes advanced knowledge estates and helps a multi-agent utility system. Information platforms are sticky, however we consider market forces are conspiring to create pressure on current enterprise fashions.
On this Breaking Evaluation, we welcome Fivetran Inc. Chief Government George Fraser. Fivetran is a foundational software program firm that has a front-row seat and distinctive visibility on knowledge flows, measurement of information, sources of information, how knowledge is getting used and the way it’s altering. On this episode, we take a look at the thesis that a lot of the analytics work being carried out on knowledge platforms dangers being commoditized by adequate, price efficient tooling, which is forcing at this time’s MDS leaders to evolve.
Clay Christensen’s idea all the time rears its head
Many people in our viewers are conversant in the epic series of lectures by Clay Christensen at Oxford College the place he so brilliantly described his idea of disruptive innovation in compellingly easy phrases.
For these not conversant in the lectures, right here’s the TL;DR:
Christensen defined his mannequin within the context of the metal trade, the place traditionally, most metal was made by built-in metal mills that price $10 billion to construct on the time. However these items known as mini-mills emerged that melted scrap steel in electrical furnaces and will construct metal for 20% lower than the built-in mills.
Mini-mills grew to become viable in late Sixties. As a result of the standard of metal constituted of scrap was uniformly dangerous, mini-mills went after the rebar market and the built-in mills stated, “Let the mini-mills have the crummy 7% GM enterprise,” as proven within the diagram above. By getting out of the rebar market, the built-in metal firms’ margins improved, lulling them into a way of calm.
However when the final of the built-in mills exited the rebar market within the late ’70s, costs tanked, commoditizing rebar. So the mini-mills moved upmarket the place there was a worth umbrella. The identical factor occurred with angle iron metal after which structural metal and it stored going till the built-in metal mannequin collapsed and all however one producer went bankrupt.
As Christiansen factors, out this occurred in autos with Toyota and lots of different industries. We’ve definitely seen it in laptop techniques and we predict an identical dynamic might happen within the software program trade typically and particularly the info platform stack — with some particular variations.
Making use of the metal trade instance to the fashionable knowledge stack ecosystem
Under we present a chart to symbolize the info equal of the metal trade in Christensen’s instance.
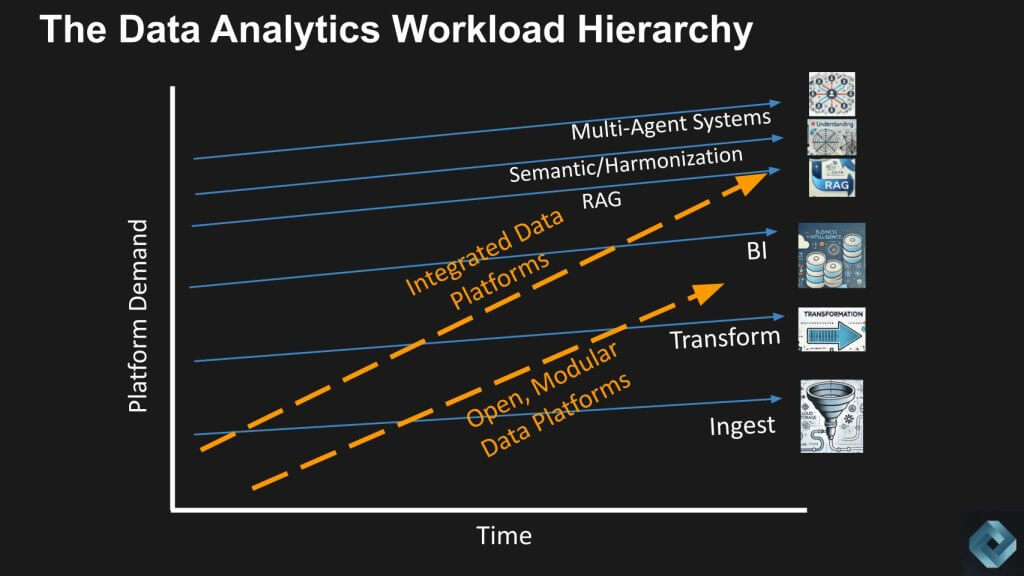
Ingest, remodel and enterprise intelligence are rebar, angle iron and structural metal, respectively. To be clear, we’re speaking in regards to the calls for on the info platform on this analogy. The connectors that extract knowledge from the applying are subtle, however the demand it locations on the system to land the info is minimal relative to the growing scale and class of at this time’s knowledge platforms.
In different phrases, for many workloads, the sophistication when it comes to distributed scale out administration capabilities is outstripping what’s wanted for many workloads. And the fashionable knowledge platform, with the intention to justify the investments of their sophistication, should transfer up the stack to new workloads.
Word: The place the analogy breaks with the metal trade is the built-in metal mills hit the ceiling of the stack – there was nowhere for them to go and so they failed in consequence. Corporations resembling Snowflake Inc. and Databricks Inc. have whole out there market enlargement alternatives that we’ll talk about intimately.
Fivetran’s distinctive perspective on the info trade
With that as background we convey George Fraser into the dialog. Fivetran is likely one of the iconic firms that outlined the fashionable knowledge stack. Snowflake, Fivetran, dbt Labs Inc. and Looker have been thought-about the 4 horsemen of the unique MDS.
This slide under from Enterprise Know-how Analysis will provide you with a way of how distinguished a place Fivetran has out there.
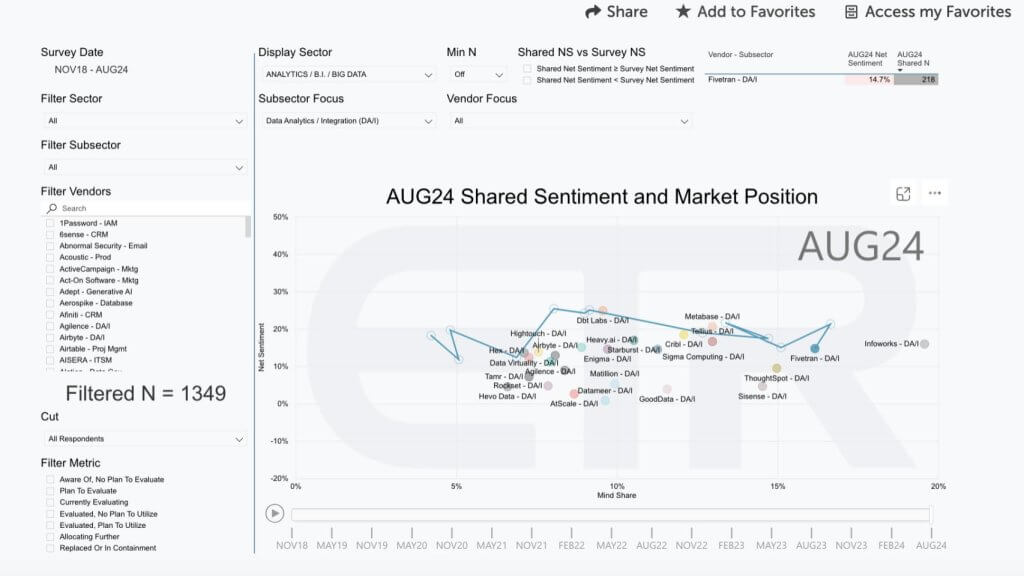
The information is from the August survey of 1,349 data know-how choice makers and reveals rising firms (privately held companies) within the knowledge/analytics/integration sector throughout the ETR knowledge set. Web Sentiment is proven on the vertical axis, which is a measure of intent to have interaction and the horizontal axis is Thoughts Share. You possibly can see the dramatic strikes that Fivetran has made since 2020 proven by that squiggly blue line.
Q1. George Fraser, is at this time’s trendy knowledge stack out over its skis with respect to the sophistication and scale of the platform relative to the overwhelming majority of workload necessities operating on the market?
I believe it’s a extremely attention-grabbing second. The information platforms are stronger than they’ve ever been, however I believe there’s a query of whether or not we’re, after a protracted interval — actually, 10 years of bundling — about to see a second of a bundling once more.
There’s an attention-grabbing indisputable fact that hangs over the whole world of information platforms, which is that almost all datasets are a lot smaller than folks notice. We see this at FiveTran, the place we’re placing knowledge into knowledge platforms. What we discover is that many of those so-called “enormous datasets” usually come from inefficient knowledge pipelines, doing issues like storing one other copy of each file each night time when the info pipeline runs.
In case you have an environment friendly knowledge pipeline, the info sizes aren’t that enormous. You additionally see that a whole lot of workloads operating on these knowledge platforms are processing many small queries quite than one large question. Proper now, we’re seeing the emergence of information lakes, that are the fastest-growing vacation spot for FiveTran — particularly Iceberg and Delta knowledge lakes.
One of many attention-grabbing implications of information lakes, whereas they’re usually considered a spot to retailer enormous datasets, in addition they produce other traits. As an example, with an information lake, you may convey a number of completely different compute entities to the identical knowledge, opening up the potential for utilizing specialised compute engines which can be extra optimized for the precise knowledge sizes most individuals work with on a day-to-day foundation.
There’s much more I might say about this, however I’ll pause right here for now. It’s an interesting second, and I believe there’s a mismatch between how these techniques get talked about and the way they’re truly utilized in follow.
The place’s all the large knowledge?
Let’s double-click on that notion of information sizes. The slide under reveals that solely 10% of the queries are greater than 100 megabytes, and solely an extra 9% go as much as 10 gigabytes. So 99% of the queries are lower than 10GB. That is largely as a result of most queries are carried out on knowledge that’s contemporary. That’s the place the worth lies. The information under comes from the weblog MotherDuck and particularly, Jordan Tigani’s “Big Data is Dead” publish. Tigani was head of product for BigQuery at Google and is CEO of MotherDuck. The information is simply a pair years previous however we consider it displays at this time’s market.
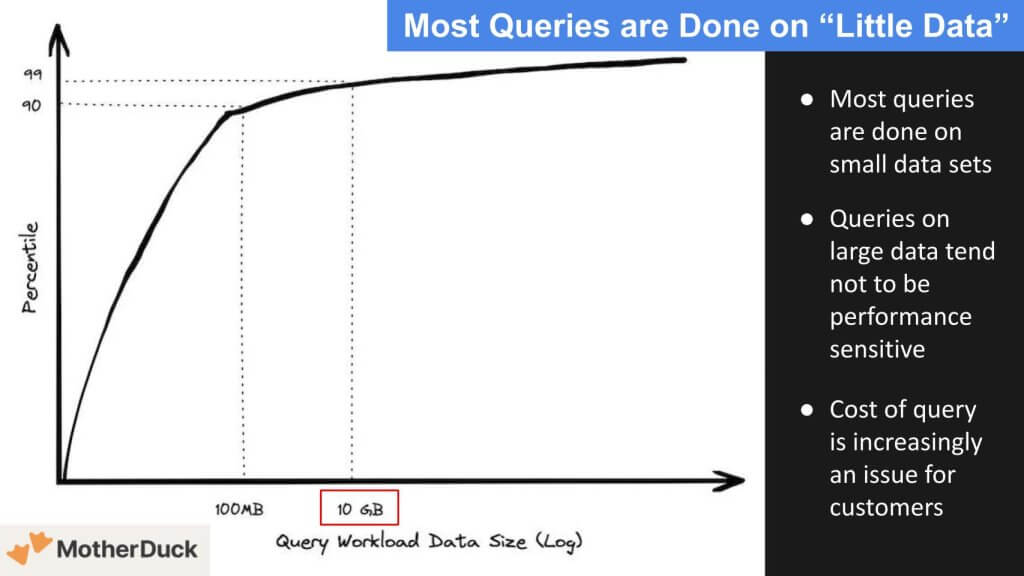
Query 2. George Fraser, are we appropriate that Jordan’s knowledge displays at this time’s actuality?
[Where’s all the big data? Listen to Fraser’s response]
Jordan has spoken lots about this, and I’ve talked to him about it as properly. I’ve additionally just lately been taking a look at Snowflake.
A couple of years in the past, Redshift truly revealed a consultant pattern of real-world queries that run, displaying what real-world question workloads seem like. The information is a bit older — Snowflake’s goes again to round 2017, and Redshift’s is a bit more current. When you can’t see the precise queries, you may view abstract statistics, together with metrics like how a lot knowledge they scanned.
What I discovered when taking a look at this knowledge was astonishing. The median question — this was true for each Snowflake and Redshift — scanned simply 64 megabytes of information. That’s exceptional! Your iPhone might theoretically deal with that question.
Now, after all, you may’t run full workloads in your iPhone, however the level is that it’s not the dimensions of the info that’s the problem; it’s the sheer quantity of queries. This aligns with what we’ve seen with our prospects at Fivetran. It opens up the chance for a unique structure sooner or later.
Think about a system with an information lake and various compute engines speaking to it.
Many of those compute engines is likely to be single-node as a result of most workloads don’t want a massively parallel processing (MPP) system. And one of many cool issues about knowledge lakes is that a number of techniques can collaborate over the identical datasets. For these uncommon, excessive workloads that require large sources to run, you may nonetheless have an MPP system take part in the identical database as different, extra optimized question engines — and even DataFrame libraries — that deal with the smaller queries, which truly make up the majority of the work folks do.
The usual EC2 occasion is bigger than most queried knowledge units
Let’s proceed exploring the present market dynamic with some extra knowledge from MotherDuck.
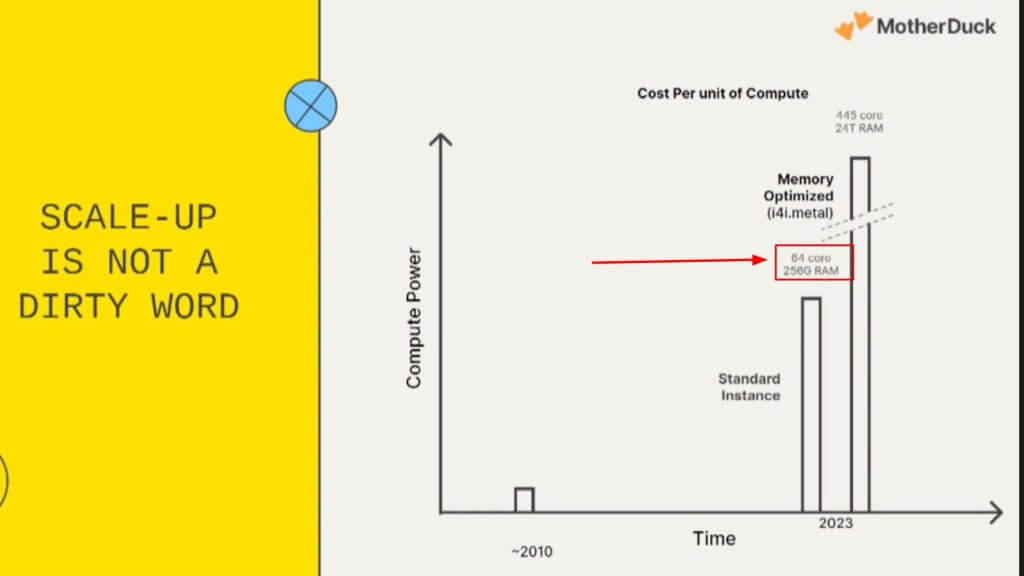
The graphic above reveals the usual occasion of AWS EC2 has 256 gigs of RAM, so you may put the whole dataset in reminiscence, that means you solely want a single node for the overwhelming majority of queries and workloads. Now to be clear, you’re not essentially doing all these queries in reminiscence, however the level is you don’t want a distributed multinode cluster to do a question for many conditions at this time.
Q3. George Fraser, you’ve analyzed current knowledge from Snowflake and Redshift about workload sizing. What does your expertise inform you about the place the prices are incurred and the scope of queries, particularly now that prospects have a selection of compute/execution engines?
[Watch Fraser discuss his research on ingest as a portion of the overall workload]
I began taking a look at this knowledge as a result of I wished to higher perceive what ingest prices are as a proportion of individuals’s whole prices.
We’ve seen examples the place, for particular person prospects, simply getting the info into the platform — ingestion — was round 20% of the full workload. That is constant throughout a broader inhabitants, which stunned me. I might’ve thought it will be a lot smaller. Nonetheless, ingest truly accounts for a big portion of what folks do on these platforms.
What does this all imply?
It’s onerous to say for certain what the long run holds, however there’s an attention-grabbing pressure embedded in these observations. I consider we’ll see extra variety in compute engines going ahead. The key platforms we use at this time aren’t going wherever, however as open codecs grow to be extra fashionable, elements of workloads will begin to peel off and use extra specialised compute engines for explicit duties.
For instance, for those who join the Fivetran knowledge lake providing at this time, we ingest the info into the info lake utilizing a service we constructed, which is powered below the hood by DuckDB. It is a nice instance of a purpose-built engine designed to do one factor extra effectively. It participates in an information lake, which is then shared with platforms like Databricks, Snowflake or no matter else the consumer must do with it.
Will specialised knowledge engines grow to be the mini-mills of the long run?
Coming again to the concept that “adequate” tooling has the potential to, just like the metal trade, commoditize the info stack.
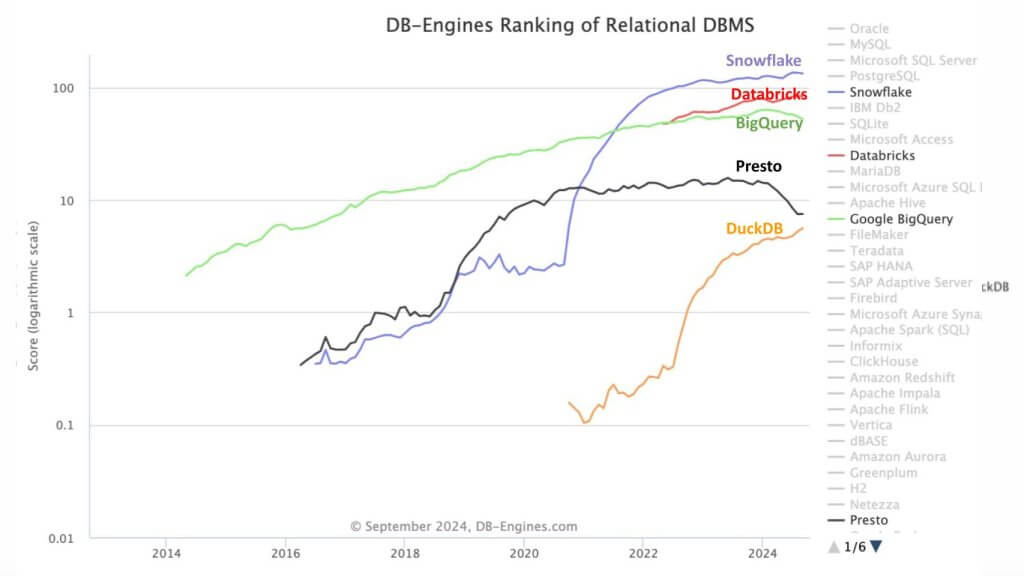
That is very current knowledge from DB-Engines. They’re the go to website for database engine reputation. What this graphic reveals is the growing reputation rating of DuckDB relative to different knowledge platforms. DuckDB has improved by nearly two orders of magnitude since 2020. The purpose is DuckDB is open-source and single-node, and in our metal analogy is the mini-mill, whereas Snowflake, Databricks and BigQuery are the built-in metal mills.
This fall. George Fraser, what are you seeing? Is there a looming shift in adoption of open-source analytic databases and do you see it as doubtlessly consuming into the recognition of built-in knowledge platforms?
[Watch and listen to Fraser’s comments that the demand for data platforms is virtually infinite]
The factor about knowledge workloads is that the demand is infinite. The dynamics of our trade are all the time much less aggressive than folks suppose. If you happen to discover a solution to do issues extra effectively, prospects will merely ask extra questions of the info.
For instance, Snowflake was very a lot a drop-in substitute for the earlier era of information warehouses. It was higher in each means and worse in none for a sure class of issues enterprises already had. It was additionally 10 instances extra cost-efficient when it comes to operating workloads. What we noticed was that individuals changed their legacy knowledge warehouses with Snowflake, however their budgets didn’t lower — they simply did extra with their knowledge.
So, to reply your query briefly: Perhaps. However understand that on this planet of information administration, as we discover extra environment friendly methods to do issues, folks simply do extra.
I might additionally add that it’s not nearly database engines. It’s additionally about issues like DataFrame execution engines. As an example, there’s a whole lot of discuss PolarDB, a really quick single-node knowledge body execution engine. There are a lot of gamers on this ecosystem, and as knowledge codecs grow to be extra open, there’s way more alternative for patrons to search out the appropriate match.
Q5. George Fraser, you have got perception into how at this time’s built-in distributors can enhance when it comes to pace and ease. Like Snowflake now with its declarative knowledge pipelines, with the incremental replace, the low-latency ingest and processing, and the way it can straight feed a dashboard. Particularly bringing that end-to- finish simplicity to maneuver the bar up when it comes to doing issues that the part elements or single node just isn’t succesful sufficient to do. In different phrases, can at this time’s MDS gamers transfer the goalpost, in a means the built-in metal mills couldn’t?
[Listen and watch Fraser’s claim that an integrated approach wins the day]
I believe for lots of shoppers, the simplicity of an built-in system will win the day. It’s important to keep in mind that the most costly factor on this planet is headcounts. You possibly can say that I can put collectively DuckDB and this and that and the opposite factor and construct a system that’s extra environment friendly mathematically. And also you’re proper. However for any explicit firm, it doesn’t take [very much] work from an engineering crew earlier than you’ve undone the entire features that you’ve got.
So there are a whole lot of prospects simply opening the field and considering what comes within the field continues to be going to be the appropriate reply just because it’s environment friendly from a folks perspective. Even when possibly there’s on the market a configuration that extra environment friendly from worth perspective.
How would possibly the MDS gamers develop their TAM?
With a purpose to justify the sophistication of at this time’s built-in knowledge platforms, we consider they need to add new performance. We’re displaying under three new components that MDS gamers might pursue, together with: 1) The addition of retrieval-augmented era; 2) A harmonization layer; and three) Multi-agent techniques. These are capabilities that companies like Snowflake and Databricks are engaged on or have a possibility by means of partnerships, to soak up the built-in simplicity that we consider is overshooting most of the conventional workloads on the backside layers.
Importantly, compared to the metal trade analogy, built-in metal mills couldn’t transfer up the stack.
Nonetheless, within the knowledge platform world, if you’d like all the info to talk the identical language — as an illustration, within the BI world, that will be metrics and dimensions — it turns into way more advanced whenever you wish to do that throughout your total utility property. The aim is to make sure the info has the identical that means it doesn’t matter what analytics or functions are interacting with it. That’s a sophisticated problem to unravel and an actual alternative to convey new worth.
We’ve mentioned this on our reveals with Benoit Dageville at Snowflake. We’ve additionally had conversations with Molham Aref from Relational AI and Dave Duggal from EnterpriseWeb. It’s nearly like we’re shifting towards a brand new form of database that capabilities as an abstraction layer. As knowledge platforms transfer up this layer, they supply built-in simplicity for utility builders, enabling them to work extra effectively.
This additionally ties into RAG
Proper now, retrieval-augmented era is utilizing a big language mannequin to make sense of various chunks of information, nevertheless it additionally wants a semantic layer to be really efficient. With out diving into the technical particulars, that is what’s known as GraphRAG. The following layer above this on the chart is when LLMs can truly take motion and invoke instruments with out being preprogrammed for each step. That is the place brokers come into play, and also you want a multi-agent framework to arrange what is actually an org chart of a military of brokers.
These are all layers that at this time’s utility platforms can evolve into, in contrast to the metal mills in our analogy. That is what we’ll proceed to discover within the coming months and years as we monitor how the applying platform evolves.
What the clever functions stack would possibly seem like sooner or later
Let’s now revisit how we envision the fashionable knowledge and utility stack evolving to assist clever knowledge apps.
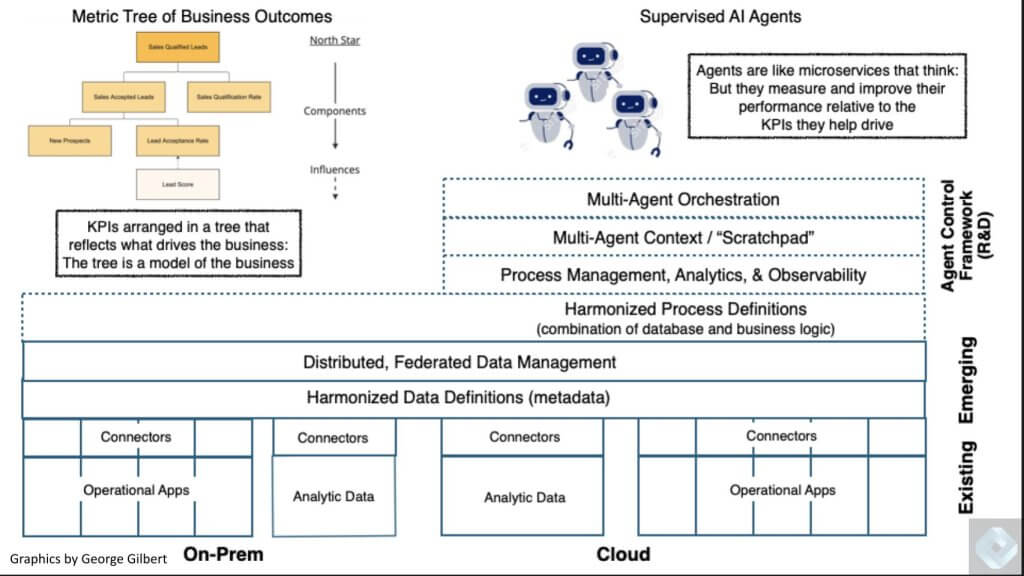
You could bear in mind final week and some episodes again on Breaking Evaluation, we laid out a vision of the evolving intelligent data applications stack. A key level here’s a lacking hyperlink is the harmonization layer, typically known as the semantic layer.
And within the higher proper is a brand new, but to be created piece of actual property that represents a multi-agent platform. We’ve reported that companies resembling Salesforce Inc., Microsoft Corp. and Palantir Applied sciences Inc. are engaged on or evolving to include a few of this new performance.
However they’re confined to their respective utility domains — whereas companies resembling UiPath Inc., Celonis Inc. and different rising gamers we’ll discuss have a possibility to transcend single utility domains and construct horizontal, multi-agent platforms that span utility portfolios and unlock trapped worth.
So it’s not solely a matter of Snowflake and Databricks going at it. There are others now within the combine as these companies aspire to be platforms for constructing clever knowledge apps.
Automation gamers will vie for agentic value-add
Under we have now an eye fixed chart from Perception Capital that reveals a few of these rising gamers throughout the agentic house. We don’t count on you to learn this, however the level is there are lots of potential gamers right here that may add worth as companions, M&A targets or opponents. There are armies for current and new companies springing as much as compete for this necessary layer.
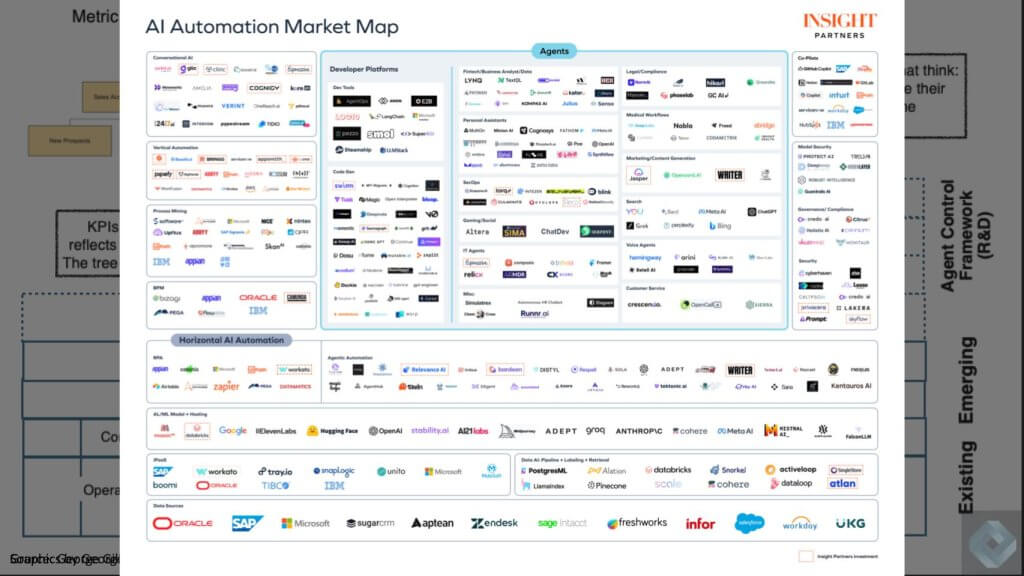
The definition of an utility for many years was the database and the info mannequin. The processes, the workflow, that was the applying logic, after which the presentation logic. And that shaped all these islands of automation. The thought of redefining this difficult discipline with a harmonization layer is that you simply summary all these islands after which you may put an agent framework round it, That agent framework will permit all these personas or functionally particular or specialised brokers to collaborate in a bigger enterprise large context, mapping to prime down objectives and executing in a backside up vogue.
We see this as the large problem and alternative for synthetic intelligence return on funding over the following 5 to 10 years.
Q6. George Fraser, maybe you may touch upon how the dialogue at this time feeds into the concept that, whereas these trendy knowledge platforms are sticky, there’s some pressure that we’ve highlighted right here which means that knowledge lakes is likely to be reworked in a means that many individuals should not speaking about.
I believe one of many non-obvious implications of the emergence of information lakes is that there will probably be extra variety of execution engines. We may even see some workloads get pulled away from the built-in knowledge platform, however on the identical time, there’s all the time new issues to do, like most of the issues that you simply simply talked about.
Disclaimer: All statements made concerning firms or securities are strictly beliefs, factors of view and opinions held by SiliconANGLE Media, Enterprise Know-how Analysis, different visitors on theCUBE and visitor writers. Such statements should not suggestions by these people to purchase, promote or maintain any safety. The content material introduced doesn’t represent funding recommendation and shouldn’t be used as the idea for any funding choice. You and solely you might be liable for your funding selections.
Disclosure: Lots of the firms cited in Breaking Evaluation are sponsors of theCUBE and/or shoppers of Wikibon. None of those companies or different firms have any editorial management over or superior viewing of what’s revealed in Breaking Evaluation.
Picture: theCUBE Analysis/Microsoft Designer
Your vote of assist is necessary to us and it helps us hold the content material FREE.
One click on under helps our mission to offer free, deep, and related content material.
Join our community on YouTube
Be a part of the neighborhood that features greater than 15,000 #CubeAlumni specialists, together with Amazon.com CEO Andy Jassy, Dell Applied sciences founder and CEO Michael Dell, Intel CEO Pat Gelsinger, and lots of extra luminaries and specialists.
THANK YOU

Source link